The short version
Our beliefs about society are largely based on information we encounter through media. This happens more and more in an “unbundled” form: Single news items are distributed through sharing on social media, sorted by algorithms, and encountered on platforms on which they were not originally published. Traditionally, this has been described with metaphors of “echo chambers” and “filter bubbles”, communities of people that are only exposed to information they agree with, leading to increasing polarization of society, and to a lack of diversity in people’s (virtual) communities. But a growing body of evidence suggests that these metaphors are misleading. In fact, as recent discussions on so-called “fake news” illustrate, biased and/or extreme information does not circulate in isolated bubbles, but instead spreads from niche communities into mainstream media and politics. NEWSFLOWS develops an alternative model of how information spreads in today’s media ecosystem – a model based on so-called feedback loops, where the output of a system influences its input. To illustrate: If a news item receives many shares on social media, this may let a recommendation algorithm show it to even more users (and journalists and politicians), making it more likely that they will act on it, again increasing the number of shares, etc. Crucially, neither the algorithm, nor the users, nor the writers alone determine the eventual spread, but a combination of their influences and feedback loops. Theoretical models and empirical methods to study such feedback loops in the social sciences and humanities are scarce. NEWSFLOWS extends innovative methods as online field experiments, data donations, and automated content analysis to conduct such studies. This will greatly enhance the theoretical understanding of news flows, but also enable media organizations to develop products conforming to calls for “responsible AI”.
The longer version
Many contemporary problems societies face are directly related to stark differences in the beliefs1 their members hold. For instance, in spite of large scientific consensus regarding human influence on climate change, many believe the opposite. Similarly, a large group came to believe that vaccination causes autism; and yet others believe in conspiracy theories about immigration. Most importantly, it seems that beliefs that may be considered extreme are held by an increasing number of people.
But how do citizens form their beliefs about societal topics? This can happen via friends, family, school – but ultimately, modern societies depend on media as a source of information that helps shaping beliefs. In fact, it is commonly argued that due to the workings of journalistic media, most citizens used to hold a socalled “common core” of beliefs they shared. However, in today’s world of social media, semi-professional niche media, and direct access to primary sources, journalistic gatekeeping is often circumvented, and the flow of information based on which citizens form beliefs has shifted [1][2]. In particular, both news sharing and algorithmic recommendations have become major forces that influence the flow of news. Due to this shift, traditional models are hard-pressed to help us understand the influence of the media on the shaping of beliefs.
To explain the role contemporary media play in shaping beliefs, two new metaphors have become extremely popular: so-called “echo chambers” and “filter bubbles”. They postulate that people are increasingly exposed to like-minded information only, leading to a lack of diverse opinions and consequently to polarization of society. However, a substantive body of recent research has shown that these metaphores are not only overly simplistic [3], but even plainly wrong. Bruns [4] argues that they distract from real problems, as supporters of extreme ideologies, instead of locking themselves up, “actively exploit the very absence of echo chambers and filter bubbles in everyday communication: drawing on human and automated means, they attempt to engineer widespread social endorsement and sharing of their populist messages […] to ensure that these messages travel far beyond the partisan in-group of the already converted” (p. 108).
Aiming for a theoretical breakthrough that isolated studies could not achieve, NEWSFLOWS addresses the need to overcome the popular yet misleading models of “echo chambers” and “filter bubbles” and replaces them by a modern, theoretically sound and empirically tested, model of news flows. Existing models are not sophisticated enough to explain how contemporary news media shape beliefs, and misrepresent their influence on societal outcomes like polarization and diversity. NEWSFLOWS will prevent that policy decisions and academic studies are based on wrong assumptions of the workings of the media system, and will provide scholars, policy makers, and practitioners alike with the models and tools they need to make well-informed decisions about how to study, regulate, and design news environments and platforms. In order to do so, we will model the flow of news across different domains and entities. News, here, encompasses any current-affairs related information of very different provenance and quality: popular media, high-quality media, but also content from non-mainstream sources (often referred to as “fringe media”), including extremist sites and so-called “fake news”.
Crucially, news flows in the modern ecosystem do not follow a linear process: If a link to a news story is shared on social media, it is seen by more people, which in turn increases future sharing. Simultaneously, it may also increase the likelihood of being seen by a journalist, who may write another story about it, which again might be shared, and so on. Similarly, if a news recommender system recommends a story to many people, it may be read more often, which again provides a signal for future recommendations.
Such situations can be described as feedback loops, as “parts joined so that each affects the other” [5](p. 54). To give a simple example: If the position where some news item is displayed on a website influences whether someone clicks on it, and if this very click again influences the position of the item for future visitors, then this constitutes a feedback loop. We distinguish between manual feedback loops (driven by active behaviour such as people sharing news with their followers) and algorithmic feedback loops (driven by algorithmic inferences based on often implicit signals). We ask:
RQ1 How do manual sharing feedback loops and algorithmic feedback loops interact?
RQ2 How do feedback loops shape news flows between fringe media, mainstream media, and politics?
RQ3 How do feedback loops influence individual beliefs?
RQ4 How do feedback loops influence polarization and diversity in different contexts?
The relevance of social media platforms and recommendation algorithms for the dissemination of journalistic content will only grow in the next decade. As emerging discussions about responsible artificial intelligence [6] highlight, platforms and algorithms are not inherently “good” or “bad”. Instead, normative values (e.g., presenting a diverse set of voices) can be build into their design [7]. The insights gained in NEWSFLOWS will allow stakeholders to do so.
Work Packages
NEWSFLOWS studies feedback loops in five interrelated work packages. WP0 (main contributor: Damian Trilling) is an overarching work package that aims at theory development and integration of the insights from the other work packages. WP1 (main contributor: Zilin Lin) will take a perspective that is focused on the news consumer and investigate feedback loops in the interplay of manual news sharing and algorithmic recommendations. WP2 (main contributor: Mónika Simon) takes a content-focused aooroach and investigates feedback loops in cross-domain flows. WP3 (main contributor: PhD candidate, to be hired in 2023) will focus on the interaction between feedback loops and beliefs. WP4 9Main contributor: Postdoc, to be hired in 2023) will investigate how the effects and relationships found in WP1–WP3 are contingent on differnet contexts such as platform characteristics, date/time, and media system.
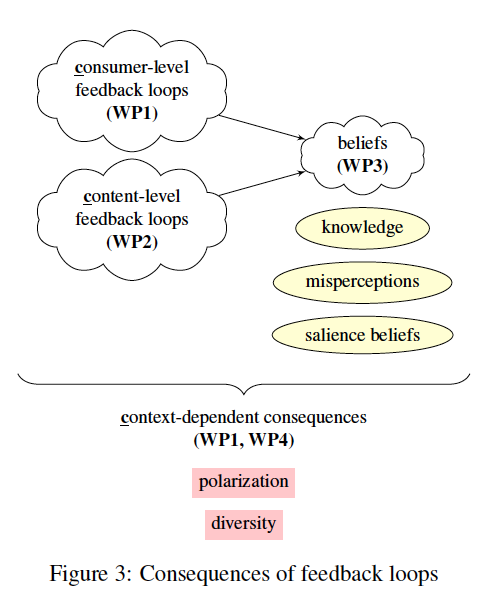
References
@article{Thorson2016,
author = {Thorson, Kjerstin and Wells, Chris},
doi = {10.1111/comt.12087},
issn = {10503293},
journal = {Communication Theory},
keywords = {10,1111,12087,algorithms,and,comt,doi,incidental exposure,media effects,one-step flow,online news,selective exposure,social media,sorts of messages to,two-step flow,what factors determine the,which citizens are exposed},
number = {3},
pages = {309--328},
title = {{Curated flows: A framework for mapping media exposure in the digital age}},
volume = {26},
year = {2016}
}
@book{Bruns2018,
address = {New York, NY},
author = {Bruns, Axel},
publisher = {Lang},
title = {{Gatewatching and news curation: Journalism, social media, and the public sphere}},
year = {2018}
}
@article{ZuiderveenBorgesius2016,
author = {{Zuiderveen Borgesius}, Frederik J and Trilling, Damian and M{\"{o}}ller, Judith and Bod{\'{o}}, Bal{\'{a}}zs and de Vreese, Claes H. and Helberger, Natali},
doi = {10.14763/2016.1.401},
journal = {Internet Policy Review},
keywords = {filter bubble,personalisation,selective exposure},
number = {1},
title = {{Should we worry about filter bubbles?}},
volume = {5},
year = {2016}
}
@book{Bruns2019,
address = {Cambridge, UK},
author = {Bruns, Axel},
publisher = {Polity},
title = {Are filter bubbles real?},
year = {2019}
}
@book{Ashby1956,
address = {London, UK},
author = {Ashby, W. Ross},
publisher = {Chapman {\&} Hall},
title = {{An Introduction to Cybernetics}},
url = {http://pcp.vub.ac.be/books/IntroCyb.pdf},
year = {1956}
}
@article{Dignum2018,
author = {Dignum, Virginia},
doi = {10.1007/s10676-018-9450-z},
issn = {1388-1957},
journal = {Ethics and Information Technology},
keywords = {brexit,government,opinion mining,petition,political participation},
number = {1},
pages = {1--3},
title = {{Ethics in artificial intelligence: Introduction to the special issue}},
volume = {20},
year = {2018}
}
@article{Helberger2019a,
author = {Helberger, Natali},
doi = {10.1080/21670811.2019.1623700},
issn = {2167-0811},
journal = {Digital Journalism},
keywords = {a threat to the,advance val-,and,are algorithmic news recommenders,democratic,how,if so,opportunity,or are they an,role of the media,to be designed to,would news recommenders need},
publisher = {Routledge},
title = {On the Democratic Role of News Recommenders},
volume = {online first},
year = {2019}
}